At the height of the Euro debt crisis, in the aftermath of the GFC, financial services risk professionals around the world were faced with the prospect of one or more countries crashing out of the Euro.. Regulators were more interested in system stability than the fortunes of a specific institution. Banks “at risk” were probably focused on protecting liquidity and projecting confidence. In my area of payments, our task was to figure out how to “turn off the payments tap” as quickly as possible for those banks declared a failed institution, while still honoring our underlying obligations to other counterparties. Irrespective of who’s interests you were representing, everyone was asking What if questions. What if two or more countries leave? What about individual banks? Which banks were most at risk of failing? What were the likely contagion effects? Different perspectives, but all starting with the same phrase: “What if…?”
Understanding the Problem
Shell plc is often credited with developing the scenario planning approach to dealing with “What if” questions in the late 1960s1. It’s an approach that is now relatively common business practice. In some industries like financial services, it is a regulatory requirement, in other industries, particularly capital-intensive ones like energy, mining and aerospace, it just makes good business sense. The formula is relatively straightforward: i) develop potential scenarios of what your operating environment may look like at some point in the future, ii) assess the likelihood of this future scenario eventuating and iii) assess the likely impact. This then sets down markers to enable an organization to create plans for how it may respond to such a future. As the old adage states: “Failing to plan is planning to fail.” Unfortunately, we don’t have a crystal ball, so how can we plan if we don’t know what the future has in store for us? This is where scenario analysis comes into its own.
In the past, accessing and analysing the detailed data required to understand the implications of these type of What-If questions was difficult, time-consuming and expensive. Consequently, many organizations relied heavily on aggregated snapshot data with a healthy dose of expert judgement. The result was another old adage: “There are two types of forecast; lucky and wrong.” Today we have access to both vast quantities of data and the expert analytical tools like process mining to make the whole process of scenario analysis far more rigorous and robust.
So How Do You Calculate the Likelihood and the Impact?
While the scenarios above appear to be quite strategic and the preserve of senior stakeholders, every manager and team leader asks What-If type questions everyday. For example:
Cassie: “How many people do we need in the contact center next Monday?”
Vivek: “Well it depends...”
In this case, the key driver for the uncertainty may be the success of an upcoming sales campaign. If it’s very successful they will need more resources to handle the leads than if the campaign falls flat. They may create a best, expected and worst case for the success of the sales campaign and then plan the resource level for each scenario.
The obvious question is: “Where do you get the data from to determine the likelihood of each case and its potential impact on resourcing?” Comparing actual, historical process event data incorporating previous sales campaigns can serve as the source for calculating the relevant probability distributions. These can then be input into a process simulation tool to determine the appropriate level of resourcing. Beyond sales campaigns, the combination of process mining and process simulation is particularly helpful when it comes to understanding the resource impact of a process change, the impact of a seemingly random demand pattern or a systems outage, or the likelihood and impact of a key control failing. Armed with this intelligence, management can then create a plan aligned to each scenario. For example, if your food delivery service is normally reliable, except when there’s a major sporting event close by, you can adjust your service offer on match days.
Back to Strategic Scenario Analysis
While the two examples discussed so far stretch from the strategic to the everyday, the connection runs deeper than a desire to answer a What-If type question. In financial services it is a regulatory requirement to calculate the capital required to cover all operational losses up to the 99.9th percentile2. Teams of experts or the regulators define the scenarios and then organizations and their lines of business work their way through these scenarios trying to assess the potential impact and likelihood of events where there may be no organizational history. Which brings us back to how do we calculate the likelihood and how do we calculate the impact of events we may not have experienced before? Typically, organizations turn to external data sources, analyzing incidents that peers may have experienced or for more novel scenarios they may revert to the price of catastrophe bonds to gain insight, or they may turn to expert panels to address these questions. However, with major events, it’s rare that its root cause can be attributed to just one failure point. It is typically a confluence of multiple, interrelated failed controls. Ariane Chapelle in her book on Operational Risk Management1 discusses the use of Fault Tree Analysis common in high-risk industries such as aerospace and nuclear energy. As a technique it decomposes a major event into the subsystems and their associated controls that contribute to the potential failure. In many cases these are everyday controls, where the failure rate is more likely to be known or at least derived from existing data. Multiplying the likelihood of the relevant sub-system control failure rates together then provides an indication of the likely probability of the major event.
Ariane provides a cybersecurity example of an employee selling confidential data on the dark web. For this event to materialize, the control to ensure the organization only hires honest staff has failed, the control to manage who has access to confidential data has failed, the control to prevent unauthorized data export, e.g., locked USB ports has failed, and ultimately there is a risk (albeit in this scenario a low probability risk) that the employee is unable to find a buyer. The failure rates (with the exception of “finding a buyer”) can all be determined through analyzing internal process data. The product of these individual probabilities will provide a reasonable assessment of the likelihood of an employee committing this crime. Multiply the probability by the number of employees and you have the exposure.
Stress Testing and Digital Twins
One of the challenges of scenario analysis is knowing when to stop asking What-If. Every insight seems to lead to another What-If question. Trying to respond manually with more expert opinion or by creating another version of a high-level model rarely reflects the reality of how processes behave under different conditions. Stress testing the impact of extreme events, i.e., black swan events is a case in point. By combining the decomposition aspect of fault tree analysis with another adage: “Don’t waste a crisis”, even extreme events can be simulated at a process level. Take for example system outages, cybercrime incidents or the recent pandemic. Each of these placed processes under stress and the event data thrown off contains powerful insights into how processes will respond under extreme conditions. Using this data as the basis for simulation makes answering yet another What-If question faster, less resource intensive and, more importantly, more realistic. In essence, a process digital twin.
Taking this to the next level and analyzing multiple processes in granular detail allows the analyst to identify cross-process correlations and develop insights into how customers will be impacted? Will they have to wait longer, will quality deteriorate? Will all services be available? It will also help understand how the demand for resources will change under specific scenarios, who will need to be re-purposed or re-skilled? The obvious conclusion is the development of the organization-wide, digital twin, but once again connecting high level scenario modeling concepts with granular, process driven insights. This approach is relevant whether it’s planning a digital transformation or understanding the risks associated with climate change or a geo-political shift in the rules-based order.
Where to Start?
As with most things, the answer is to start small and expand in line with knowledge, experience and resources. Start with an existing process and ask the type of questions Cassie and Vivek were dealing with. Try to remove a process step, increase the volume, reduce the resourcing, simulate, and see what happens! The power of process mining together with process simulation is providing a common toolset to support business as usual What-If questions. But from there the journey quickly gathers momentum, right up to developing digital twins and addressing complex stress test scenarios all in the same package.
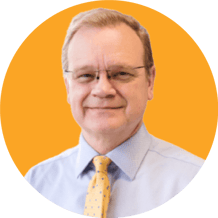